The Evolutionary Journey of Artificial Intelligence: From Inception to Societal Impact
Artificial Intelligence (AI) has experienced remarkable progress since its inception, revolutionizing various facets of our lives. This article delves into the journey of AI development from its early years to the present day, highlighting pivotal milestones and its profound impact on society and the economy.
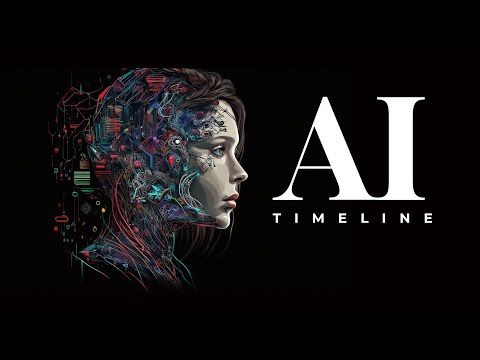
Early Beginnings:
The roots of Artificial Intelligence (AI) can be traced back to the seminal Dartmouth Conference in 1956, where the term "artificial intelligence" was first coined. This conference, organized by John McCarthy, Marvin Minsky, Nathaniel Rochester, and Claude Shannon, marked the beginning of systematic AI research.
Key figures such as Alan Turing and John McCarthy played instrumental roles in shaping the early landscape of AI. Turing's concept of the Turing Test, proposed in his seminal paper "Computing Machinery and Intelligence," laid the foundation for assessing a machine's ability to exhibit intelligent behavior comparable to that of a human. McCarthy, often referred to as the "father of AI," introduced the Lisp programming language and developed the concept of "logical AI," emphasizing the use of symbolic logic for problem-solving.
During this nascent stage, early AI programs primarily focused on rule-based systems and symbolic reasoning. One notable example is the Logic Theorist, developed by Allen Newell, J.C. Shaw, and Herbert Simon in 1956, which aimed to prove mathematical theorems using symbolic logic. However, these early AI programs faced significant limitations, primarily due to the lack of computational power and the complexity of modeling human cognition.
Despite these challenges, the exploration of early AI laid the groundwork for subsequent advancements. It provided valuable insights into the fundamental principles of intelligence and paved the way for the development of more sophisticated AI techniques in the years to come.
Growth and Expansion:
Expansion of AI Research and Applications:
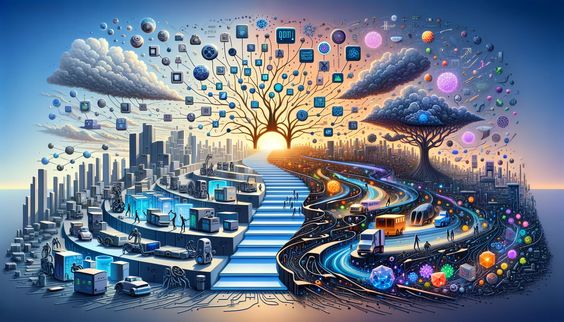
Emergence of Modern AI:
Advent of Neural Networks and Deep Learning:
Landmark Achievements:
Role of Big Data and Computational Power:
Societal Impacts:
Impact on Various Sectors:
Ethical Considerations:
Positive Contributions:
Economic Implications:
The widespread adoption of Artificial Intelligence (AI) is reshaping economies, with profound implications for labor markets, productivity, and business dynamics.
Effect on Labor Markets and Productivity:
Potential for New Industries and Business Opportunities:
Challenges Related to Income Inequality and the Digital Divide:
In conclusion, the economic implications of AI adoption are vast and multifaceted, encompassing changes in labor markets, productivity, and business dynamics. While AI has the potential to create new industries and business opportunities, it also presents challenges related to income inequality and the digital divide. Addressing these challenges requires concerted efforts from policymakers, businesses, and civil society to ensure that the benefits of AI are shared equitably and inclusively.
Future Outlook:
The future trajectory of Artificial Intelligence (AI) development holds great promise, with potential advancements in key areas such as AI ethics, explainability, and general intelligence shaping the evolution of the technology
.
Advancements in AI Ethics:
Enhanced Explainability:
Progress in General Intelligence:
Importance of Responsible AI Governance:
In conclusion, the future of AI development holds immense potential for advancements in areas such as AI ethics, explainability, and general intelligence. Responsible AI governance and collaboration between stakeholders will be essential in guiding the ethical development and deployment of AI technologies, ensuring that they benefit society while minimizing potential risks and challenges.
Conclusion
The evolution of AI has been marked by significant progress, from its humble beginnings to its current status as a transformative technology. As AI continues to evolve, it is essential to consider its societal and economic impacts and work towards harnessing its potential for the benefit of humanity.